PENALTY REWARD ANALYSIS (PRA)
FACTORS ON CUSTOMER SATISFACTION AND THEIR IMPACT
With driver analyses such as the penalty reward analysis, we we use quantitative market research to find out which factors influence the satisfaction of your customers in which way. We often use penalty reward analysis (PRA) in the areas of customer feedback, customer satisfaction, customer experience consumer empathy, but also in employee satisfaction studies. After a driver analysis, the drivers are divided into different categories based on their mode of action. Through this multivariate analysis, you can take targeted measures to increase customer satisfaction and purchase intent and thus be successful in the long term. Typical questions you can answer with a penalty reward analysis:
- How is a feature influenced by one or more other features?
- Are certain product features or services features hygiene factors or delighter factors?
- What factors should be prioritized to improve customer satisfaction?
- How can budget decisions be made in relation to these factors?
THE KANO MODE OF CUSTOMER SATISFACTION
The relationship between features is not always linear, as is assumed in many other procedures (e.g., regression analysis, structural equation modeling). According to the Kano Model of Customer Satisfaction three types of drivers can be distinguished:
- Basic drivers
There are expectations regarding a minimal standard. If these expectations are not fulfilled it leads to dissatisfaction. But if the hygiene factors are met, it does not lead to satisfaction. - Delighters
Regarding this driver there are no expectations. If it performs, nevertheless, satisfaction is achieved. But if the delighter does not perform there are no consequences. - One-dimensional driver
Both satisfaction and dissatisfaction can occur according to the degree to performance.
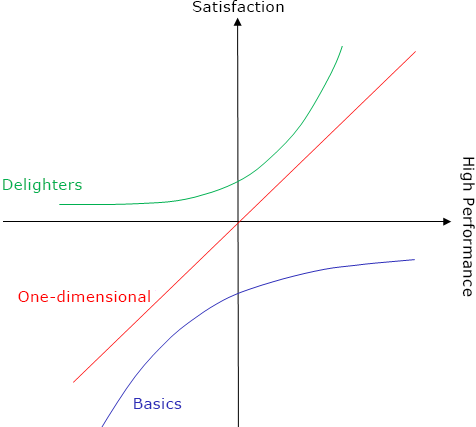
Penalty Reward Analysis: Types of Drivers according to Kano
HOW PENALTY REWARD ANALYSIS WORKS
These two basic prerequisites must be met for the variables in order for a penalty reward analysis to be carried out:
- The relationships between the independent variables and the dependent variable must be positive. Otherwise, recoding is necessary. If this is not the case, this requirement can be fulfilled with a simple recoding.
- The Penalty Reward procedure divides the sample into three groups, “high”, “middle” and “low”, according to the levels of the dependent variable. The “high” and “low” groups must not be empty.The independent drivers are each converted into their own dichotomous 0-1 pair of variables (satisfied/dissatisfied) according to their scale and included together with the dependent variable in a regression model.For each driver, the Penalty Reward procedure determines how far poor ratings for this driver exclusively correspond to poor ratings for the dependent variable, i.e., a driver is penalized. Since the “penalty” can thus be unambiguously traced back to one driver, multicollinearity does not represent a problem. It is also tested how far good ratings for a certain driver exclusively correspond to good ratings for the dependent variable, i.e., a driver is “rewarded”.
The relationship arising from the amount of Penalty and Reward is decisive for determining the type of the driver. When Penalty is dominant it is a Basic Driver, when Reward is dominant it is a Delighter. When Penalty and Reward are roughly equal, it is a One-dimensional driver or a performance driver. From the relation of the sum of Penalty and Reward of a driver to the total of the sums of all drivers, the importance of the driver can also be derived.
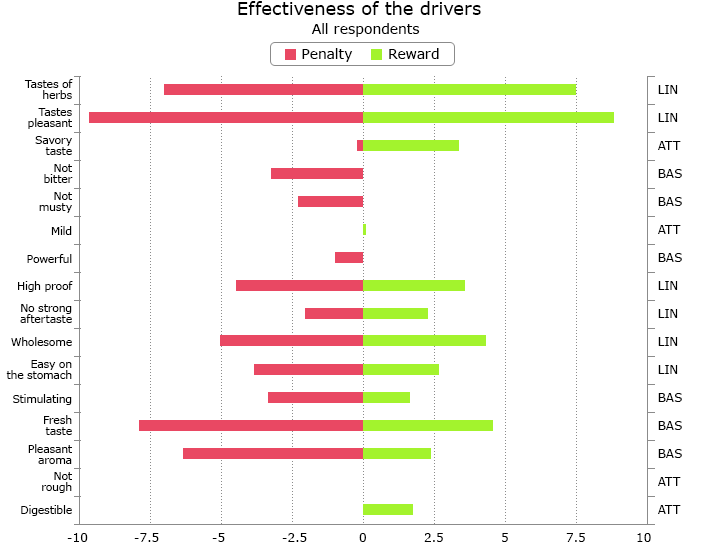
Penalty Reward analysis: Categorization of Drivers
PENALTY REWARD ANALYSIS WITH ACTION RELEVANCE MATRIX
The results of the Penalty Reward Analysis can be clearly displayed in an Action Relevance Matrix. For this purpose, the importance of the drivers on the x-axis and the mean value of the drivers on the y-axis are mapped. The driver types are distinguished by colored icons. The space is divided into four ranges by the average of all driver strengths (x-axis) and all driver averages (y-axis).
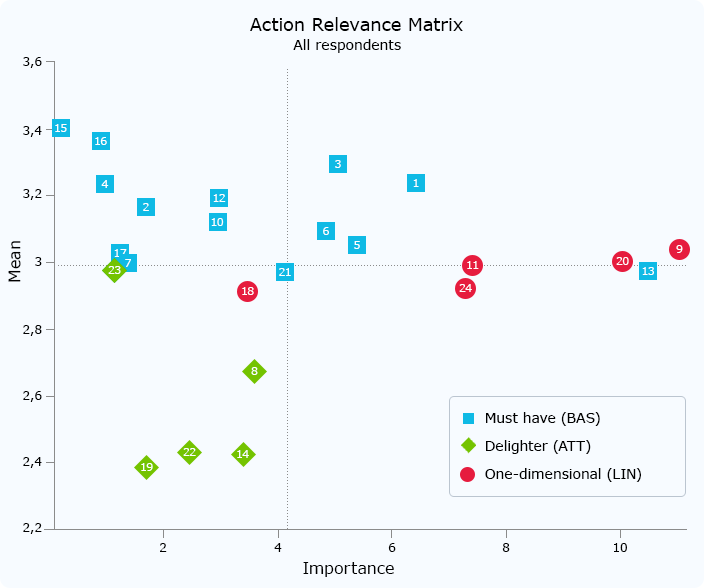
Penalty Reward Analysis: Action Relevance Matrix